About
Welcome to my page! I graduated from INSA Rouen Normandie in 2018 with a specialization in applied Mathematics. Following that, I pursued a year as a Research Engineer at INSA Rouen Normandie, collaborating with the H. Becquerel Center in Rouen on medical image segmentation. Subsequently, I embarked on a doctoral thesis at INSA Rouen Normandie focusing on medical image segmentation using hybrid variational approaches and deep learning techniques, under the supervision of Carole Le Guyader from LMI and Caroline Petitjean from LITIS. I successfully defended my Ph.D on December 9, 2022. Since January 2, 2023, I have been working as a postdoctoral researcher at CEREMA on the DEPHY3GEO project, focusing on cliff image segmentation from drone imagery. Alongside my postdoc, I am also involved in teaching activities at INSA, serving as a part-time lecturer in the Mathematics department and as a teaching assistant in the STPI department.
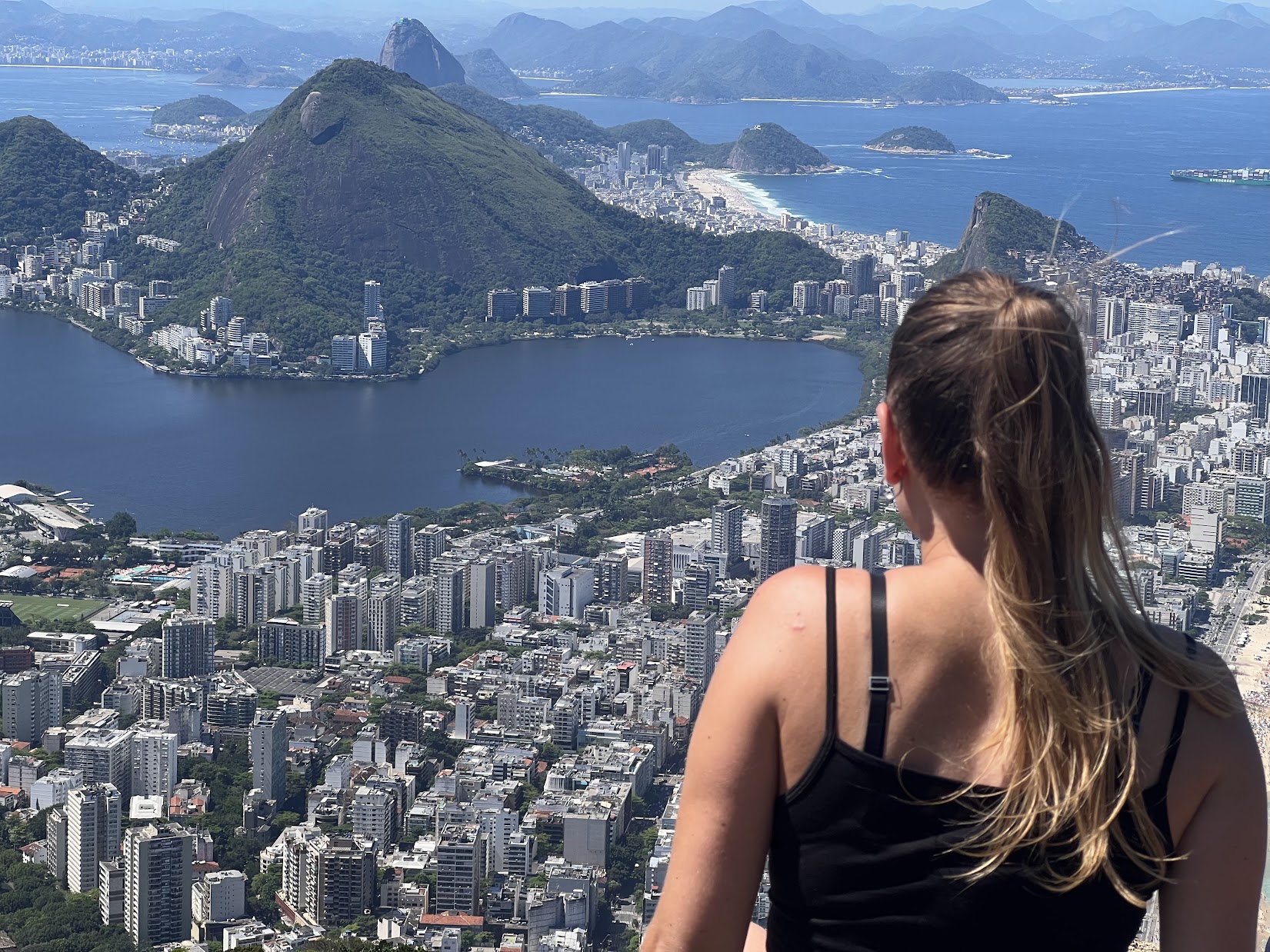
Outside of my professional activities, I love traveling. I have visited many European capitals and have been fortunate enough to embark on extensive trips to the United States 🇺🇸, India 🇮🇳, Canada 🇨🇦, and recently Brazil 🇧🇷.
Furthermore, I am deeply passionate about sports. Not only do I engage in various sporting activities, but I also have a keen interest in them. I enjoy following and discovering all sports, but I am particularly knowledgeable about biathlon and rugby 🏉 ! Above all, I love spending time with my family and friends, whether it's over a good meal, a board game session, or a nature walk.
Research
Research interests
My research interests lie in the development of advanced methods for image segmentation, particularly in the medical domain. I am particularly interested in exploring hybrid methods of variational models and deep learning to integrate geometric and topological constraints. The goal is to improve the accuracy and reliability of segmentation results while also providing more explainability to the segmentation model through clear mathematical tools. Incorporating prior knowledge into the segmentation process, such as topological prescriptions and geometric constraints, helps correct recurring issues, including aberrant errors such as holes, isolated pixels, etc.
To achieve this, I focus on designing new loss functions that include these a priori knowledge, for example, through a regularization term based on weighted total variation, volume/area penalties, or a Mumford-Shah term. I also explore innovative approaches that interpret the segmentation process as a registration task, using principles of nonlinear elasticity to ensure volume and topology preservation.
My research involves theoretical analysis of models, algorithm development, and experimental validation using medical imaging datasets, with the ultimate goal of making significant improvements over existing segmentation methods, especially in complex scenarios (poorly defined contours, limited data, imbalanced classes, etc.).
I am also interested in the segmentation of fine structures, particularly cracks in images of Normandy cliffs acquired during drone flights. Studying this type of imagery is particularly challenging due to its sensitivity to weather conditions and the lack of appropriate datasets. To address these issues, I work with thermal infrared images, which are less weather-sensitive, and I develop a public dataset for crack segmentation. I develop decomposition models based on directional total variation to extract cracks from these images. In parallel, I also study the fusion of these bi-spectral images in convolutional neural networks.
Keywords: Signal and image processing, data modeling in medical sciences and geology, convolutional neural networks, optimization, segmentation, registration, decomposition, variational methods, deep learning methods, hybrid model, geometric and topological constraints.
Resume
Below, you can find a shortened version of my resume. To access the full version, click on the "Download full Resume" button.
Education
Ph.D in Applied Mathematics
2019 - 2022
INSA Rouen Normandie (LMI), Rouen, France
- Title: Geometric and Topological Constraints for Medical Image Segmentation: Hybrid Variational and Deep Learning Approaches
- Advisors: Carole Le Guyader (LMI, INSA Rouen Normandie) and Caroline Petitjean (LITIS, Rouen University)
- Defense: 2022-12-09 in front of a jury composed of :
- Jean-François Aujol, Professor, Bordeaux University - Reviewer
- Olivier Bernard, Professor, INSA Lyon - Reviewer
- Noémie Debroux, Associate Professor, Clermont Auvergne University - Examiner
- Vincent Duval, Researcher, INRIA Paris - Examiner
- Gilles Gasso, Professor, INSA Rouen Normandie - Examiner & President
- Fabien Pierre, Associate Professor, Lorraine University - Examiner
- Carole Le Guyader, Professor, INSA Rouen Normandie- Director
- Caroline Petitjean, Professor, Rouen University - Director
- Funding: Normandy Region
Engineer degree - Applied Mathematics
2013 - 2018
INSA Rouen Normandie, Rouen, France
Mathematics and Computer Science
Professional Experience
Postdoctoral fellow
2023 - Present
CEREMA, LE Grand-Quevilly, France
- Subject: automatic detection of fractures in images of coastal cliffs, building a database of images acquired in the visible and thermal infrared
- Advisor: Dr Raphaël Antoine
- Project: DEPHY3GEO
- Research topics: image processing, crack detection, TIR, Visible, Convolutional Neural Network, Badlands
Research engineer
2018-2019
INSA Rouen Normandie, Rouen, France
- Subject: segmentation/weakly supervised learning - applications to medical images (Centre H. Becquerel, Rouen 76)
- Advisors: Professor Christian Gout, Professor Caroline Petitjean
- Project: M2SiNum - (WP : Image Processing)
- Research topics: image processing, variational methods, machine learning, deep learning, math. modelling, simulation, data science: approximation of large datasets - image processing - medical applications
Data Scientist Intern
2018
AID Add Intelligence to data, Paris, France
Keywords: time series, machine learning, deep learning, statistics
Research assistant
2016
Montréal University, Montreal, Canada
- Subject: research on a cardiac disease, focusing on analyzing data recorded from patients suffering from atrial flutter syndrome
- Advisor: Professor Alain Vinet
- Research topics: statistical analysis and development of computational models for cardiac anomalies, machine learning
Publications
Teaching
Teacher and Teaching Assistant at INSA Rouen Normandie since 2018, in the departments of Engineering Science and Mathematical Engineering.
Position and Location | Field | Level | Content | Type | Hours (eq. TD) |
---|---|---|---|---|---|
Part-time teacher, INSA Rouen | Science and Engineering | L1 | Real Functions of Several Variables | TD | 21 |
Linear Algebra | TD | 75 | |||
Mathematical Engineering | L3 | Probabilities | TD | 27 | |
M1 | Partial differential equation | CM | 18 | ||
TD / TP | 36 | ||||
DCCE, INSA Rouen | Science and Engineering | L2 | Probability calculus | TD | 149 |
Real functions of several variables (L1): 21h
This course aims to introduce analysis tools for solving problems arising from the modeling of engineering situations: differential equations, optimization, multiple integrals, and topology of normed vector spaces. I supervised tutorial sessions for groups averaging 25 L1-level students. I also participated in grading mid-term and end-of-term exams.
Keywords: linear differential equations, functions of several variables: limits and continuity, differentiability of a function on an open set of ℝn, constrained and unconstrained optimization.
Linear Algebra (L1): 37.5h
The aim of this course is to acquire basic notions related to vector spaces, to model and solve linear problems, and to define the concept of dimension. This course also aims to deepen the understanding of elementary geometry in the plane and in space. I supervised tutorial sessions for a group of 28 L1-level students. I participated in developing mid-term and end-of-term exams as well as in grading.
Keywords: vector spaces, linear transformations, matrices and determinants, reduction of endomorphisms.
Probabilities (L3): 27h
The aim of this course is to introduce the main concepts of probability theory, provide essential tools in the field of probability, and prepare for the study of statistics. I supervised tutorial sessions for groups averaging 12 students, as part of an exchange with a Moroccan school at the L3 level. I also participated in grading mid-term and end-of-term exams, as well as makeup exams.
Keywords: random variables, random vectors, sum of random variables, convolution, convergence of random variables.
Partial differential equation (M1): 54h
This course is an introduction to the theoretical and numerical study of certain Partial Differential Equations (elliptic, parabolic, and hyperbolic boundary value problems) as well as their discretizations (finite differences, finite volumes, finite elements). The objective is to relate them to their physical interpretation, establish a classification, understand their interpretation in both classical and generalized senses, know their main properties, and, whenever possible, solve them. I delivered lectures (18 hours) and supervised tutorial/practical sessions (36 hours) for 60 M1-level students. I developed and graded mid-term and end-of-term exams. I participated in the end-of-term jury.
Keywords: boundary value problems, discretization, consistency, stability, convergence, numerical schemes.
Probability calculus (L2): 149h
This course aims to deepen the high school curriculum in probability, enabling the understanding and modeling of numerous random situations using series, generalized integrals, and linear algebra. I supervised tutorial sessions for groups averaging 25 L2-level students. I participated in developing mid-term and end-of-term exams, as well as in grading. Some of these class hours were conducted in hybrid mode, with half of the class attending in-person and the other half participating remotely.
Keywords: counting, probability spaces, discrete and continuous random variables, pairs of random variables, convergence theorems.
I also had the opportunity to supervise several students during internships or projects.
• May 2022: Participation in the evaluation of a Master's level final project, accompanied by Professor Carole Le Guyader, INSA Rouen Normandie, France.
Topic: Approximation of one-dimensional signals.
• May - August 2023: Co-supervision of a specialized internship, M1 level, with Dr. Raphael Antoine, CEREMA, Le Grand-Quevilly, France.
Topic: Super-resolution and multispectral detection of fine structures.
• October 2023: Participation in the evaluation of specialized internships, M1 level, accompanied by Professor Nathalie Chaignaud, INSA Rouen Normandie, France.
• September 2023 - February 2024: Co-supervision of a Master's level final project (400 hours of work provided by the student), with Professor Christian Goût, INSA Rouen Normandie, France.
Topic: Development of a C++ library for Machine Learning.
• October 2023 - February 2024: Co-supervision of a final project, with Professor Caroline Petitjean, University of Rouen, France.
Topic: Automatic segmentation using deep learning of fine structures.
Other activities
Scientific mediation
• Participation in orientation forums for high school students.
• Participation at the welcome ceremony for Norman doctoral students.
• Participation at the "Big Data & AI" fair in Paris.
• Intervention with M2 students of the Master SID, University of Rouen.
Winners of the first Datathon Normandy 🏆
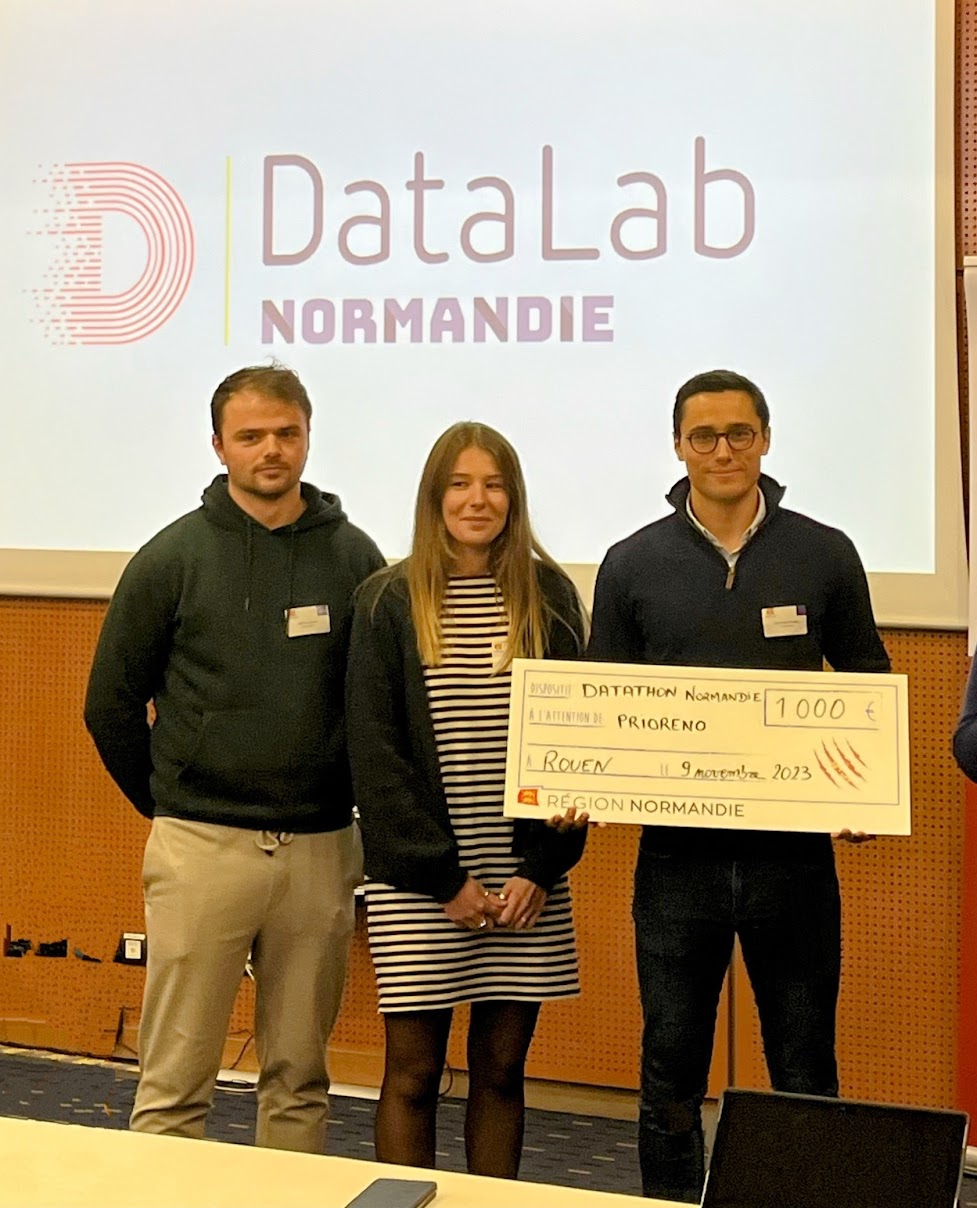
Thursday, November 9, 2023, marked the conclusion of the Normandy Datathon, a collaborative initiative between the Normandy Region and Datalab Normandy. For a whole month, participants were tasked with conceiving a prototype for an innovative digital solution leveraging open data to address challenges in energy, health, and the environment, specifically for the Normandy area.
My team, which included Martin Lambert, Jean-Eudes Rouffiac, and myself, proudly presented Prioreno. Our solution aimed to facilitate collective action in renovating Normandy's residential properties, with a focus on prioritizing those most in need. Through the development of a scoring system for energy vulnerability and an assessment of energy potential for each building, Prioreno served as a decision-making tool for stakeholders.
This decision support tool is intended for both public organizations in the Normandy region to effectively and precisely manage the energy transition of Normandy's residential real estate and to offer assistance directly to households facing energy precarity. However, it is also intended for individuals, both homeowners and tenants, who can find ways to improve the energy efficiency of their homes.
On Thursday, November 9, 2023, the jury, composed of elected officials from the region and data professionals, convened, and each team had to pitch their project in 5 minutes. Our solution, Prioreno, convinced them, and we won first place!
A big thank you to the region and the organizers!
You can find a report on the datathon by clicking on this link.
Keywords: Normandy datathon, open data, machine learning, user interface, development, AI for good !
Referee
Evaluation of an article submitted to the journal Neurocomputing (May 2023).
Administrative
Elected to the laboratory council (LMI) and the scientific council of INSA Rouen Normandie (2020-2022).
Associative
Member of the Society of Applied and Industrial Mathematics (SMAI).
Competitions and open science
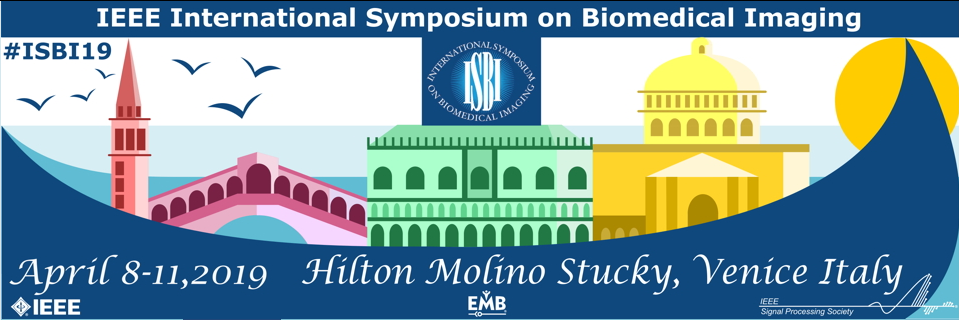
I co-organized the SegTHOR (Segmentation of THoracic Organs at Risk in CT images) challenge with Professor Caroline Petitjean (LITIS), Professor Bernard Dubray (Center H. Becquerel) and Professor Su Ruan (LITIS) at IEEE ISBI’19.
The SegTHOR challenge addresses the problem of organs at risk segmentation in Computed Tomography (CT) images. In lung and esophageal cancer, radiation therapy planning begins with the delineation of the target tumor and healthy organs located near the target tumor, called Organs at Risk (OAR) on CT images. Routinely, the delineation is largely manual which is tedious and source of anatomical errors. In this challenge, the goal is to automatically segment 4 OAR: heart, aorta, trachea, esophagus. Participants will be provided with a training set 40 CT scans with manual segmentation. The test set will include 20 CT scans.
The challenge was hosted on Codalab. Participants could submit their models directly on the website. I created the performance metrics to score the participants' models. There were over 100 submissions from different teams. The top teams came to present their models on April 8, 2019, in Venice, Italy.